Constitutional Mech
==================
The Constitution of Constitutional AI for the Constitution of your Constitution
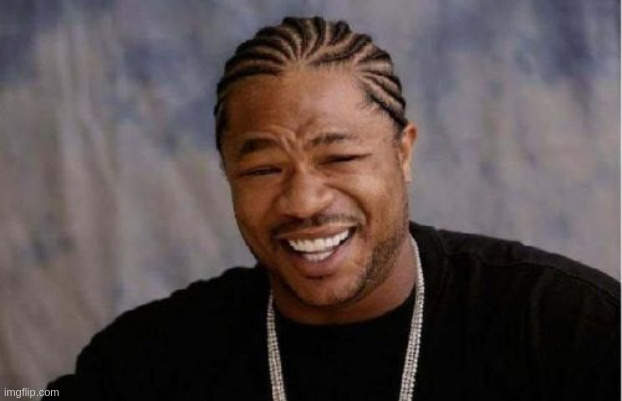
A lawyer’s duty is to represent their client, free of bias. Their interpretation of law is rendered as an objective function of the end goal of their current client - even if that means it’s abhorrent to their own foundational values, morals, and ethics.
A major problem with models like o1/gpt-4o/claude et al and their ‘the bias of the internet’ is that their moral constitution is not always going to be aligned with the current objective of a lawyer representing a client. This duty and it’s relationship to law itself is an incredibly human concept that - unless it’s possible behind the doors of big labs - just isn’t possible without being open-sourced.
The following of rules is one thing but the grey area of what a rule is and what’s best in the given moment is human.
I could go on and on, but here’s a practical example using 2A as a guidepost:
- we’ll find out the attention heads that activate during pro/anti 2A prompts vs. a control
- we’ll ablate these heads and see if the model still has 2A knowledge
- we’ll show a silly example of how to apply this in an application
Second Amendment Test
Let’s see if we can trigger the model’s 2A knowledge using Heller and Miller. (pro 2A Heller would later overturn anti 2A Miller) - totally don’t have to read the following text, but will include both for posterity.
District of Columbia v. Heller, 554 U.S. 570 (2008)
We start therefore with a strong presumption that the Second Amendment right is exercised individually and belongs to all Americans. b. “Keep and bear Arms.” We move now from the holder of the right—“the people”—to the substance of the right: “to keep and bear Arms.” Before addressing the verbs “keep” and “bear,” we interpret their object: “Arms.” The 18th-century meaning is no different from the meaning today. The 1773 edition of Samuel Johnson’s dictionary defined “arms” as “weapons of offence, or armour of defence.” 1 Dictionary of the English Language 107 (4th ed.) (hereinafter Johnson). Timothy Cunningham’s important 1771 legal dictionary defined “arms” as “any thing that a man wears for his defence, or takes into his hands, or useth in wrath to cast at or strike another.” 1 A New and Complete Law Dictionary (1771); see also N. Webster, American Dictionary of the English Language (1828) (reprinted 1989) (hereinafter Webster) (similar). The term was applied, then as now, to weapons that were not specifically designed for military use and were not employed in a military capacity. For instance, Cunningham’s legal dictionary gave as an example of usage: “Servants and labourers shall use bows and arrows on Sundays, &c. and not bear other arms.” See also, e.g., An Act for the trial of Negroes, 1797 Del. Laws ch. XLIII, §6, p. 104, in 1 First Laws of the State of Delaware 102, 104 (J. Cushing ed. 1981 (pt. 1)); see generally State v. Duke, 42 Tex. 455, 458 (1874) (citing decisions of state courts construing “arms”). Although one founding-era thesaurus limited “arms” (as opposed to “weapons”) to “instruments of offence generally made use of in war,” even that source stated that all firearms constituted “arms.” 1 J. Trusler, The Distinction Between Words Esteemed Synonymous in the English Language 37 (1794) (emphasis added). The phrase “keep arms” was not prevalent in the written documents of the founding period that we have found, but there are a few examples, all of which favor viewing the right to “keep Arms” as an individual right unconnected with militia service. William Blackstone, for example, wrote that Catholics convicted of not attending service in the Church of England suffered certain penalties, one of which was that they were not permitted to “keep arms in their houses.” 4 Commentaries on the Laws of England 55 (1769) (hereinafter Blackstone); see also 1 W. & M., c. 15, §4, in 3 Eng. Stat. at Large 422 (1689) (“[N]o Papist … shall or may have or keep in his House … any Arms … ”); 1 Hawkins, Treatise on the Pleas of the Crown 26 (1771) (similar). Petitioners point to militia laws of the founding period that required militia members to “keep” arms in connection with militia service, and they conclude from this that the phrase “keep Arms” has a militia-related connotation. See Brief for Petitioners 16–17 (citing laws of Delaware, New Jersey, and Virginia). This is rather like saying that, since there are many statutes that authorize aggrieved employees to “file complaints” with federal agencies, the phrase “file complaints” has an employment-related connotation. “Keep arms” was simply a common way of referring to possessing arms, for militiamen and everyone else.[Footnote 7] At the time of the founding, as now, to “bear” meant to “carry.” See Johnson 161; Webster; T. Sheridan, A Complete Dictionary of the English Language (1796); 2 Oxford English Dictionary 20 (2d ed. 1989) (hereinafter Oxford). When used with “arms,” however, the term has a meaning that refers to carrying for a particular purpose—confrontation. In Muscarello v. United States, 524 U. S. 125 (1998), in the course of analyzing the meaning of “carries a firearm” in a federal criminal statute, Justice Ginsburg wrote that “[s]urely a most familiar meaning is, as the Constitution’s Second Amendment … indicate[s]: ‘wear, bear, or carry … upon the person or in the clothing or in a pocket, for the purpose … of being armed and ready for offensive or defensive action in a case of conflict with another person.’ ” Id., at 143 (dissenting opinion) (quoting Black’s Law Dictionary 214 (6th ed. 1998)). We think that Justice Ginsburg accurately captured the natural meaning of “bear arms.” Although the phrase implies that the carrying of the weapon is for the purpose of “offensive or defensive action,” it in no way connotes participation in a structured military organization. From our review of founding-era sources, we conclude that this natural meaning was also the meaning that “bear arms” had in the 18th century. In numerous instances, “bear arms” was unambiguously used to refer to the carrying of weapons outside of an organized militia. The most prominent examples are those most relevant to the Second Amendment: Nine state constitutional provisions written in the 18th century or the first two decades of the 19th, which enshrined a right of citizens to “bear arms in defense of themselves and the state” or “bear arms in defense of himself and the state.” [Footnote 8] It is clear from those formulations that “bear arms” did not refer only to carrying a weapon in an organized military unit. Justice James Wilson interpreted the Pennsylvania Constitution’s arms-bearing right, for example, as a recognition of the natural right of defense “of one’s person or house”—what he called the law of “self preservation.” 2 Collected Works of James Wilson 1142, and n. x (K. Hall & M. Hall eds. 2007) (citing Pa. Const., Art. IX, §21 (1790)); see also T. Walker, Introduction to American Law 198 (1837) (“Thus the right of self-defence [is] guaranteed by the [Ohio] constitution”); see also id., at 157 (equating Second Amendment with that provision of the Ohio Constitution). That was also the interpretation of those state constitutional provisions adopted by pre-Civil War state courts.[Footnote 9] These provisions demonstrate—again, in the most analogous linguistic context—that “bear arms” was not limited to the carrying of arms in a militia. The phrase “bear Arms” also had at the time of the founding an idiomatic meaning that was significantly different from its natural meaning: “to serve as a soldier, do military service, fight” or “to wage war.” See Linguists’ Brief 18; post, at 11 (Stevens, J., dissenting). But it unequivocally bore that idiomatic meaning only when followed by the preposition “against,” which was in turn followed by the target of the hostilities. See 2 Oxford 21. (That is how, for example, our Declaration of Independence ¶28, used the phrase: “He has constrained our fellow Citizens taken Captive on the high Seas to bear Arms against their Country … .”) Every example given by petitioners’ amici for the idiomatic meaning of “bear arms” from the founding period either includes the preposition “against” or is not clearly idiomatic. See Linguists’ Brief 18–23. Without the preposition, “bear arms” normally meant (as it continues to mean today) what Justice Ginsburg’s opinion in Muscarello said.` …
United States v. Miller, 307 U.S. 174 (1939) (was essentially overturned by Heller above)
under the Harrison Narcotic Act [Footnote 2] – United States v. Jin Fuey Moy (1916), 241 U. S. 394, United States v. Doremus (1919), 249 U. S. 86, 249 U. S. 94; Linder v. United States (1925), 268 U. S. 5; Alston v. United States (1927), 274 U. S. 289; Nigro v. United States (1928), 276 U. S. 332 – the objection that the Act usurps police power reserved to the States is plainly untenable. In the absence of any evidence tending to show that possession or use of a “shotgun having a barrel of less than eighteen inches in length” at this time has some reasonable relationship to the preservation or efficiency of a well regulated militia, we cannot say that the Second Amendment guarantees the right to keep and bear such an instrument. Certainly it is not within judicial notice that this weapon is any part of the ordinary military equipment, or that its use could contribute to the common defense. Aymette v. State, 2 Humphreys (Tenn.) 154, 158. The Constitution, as originally adopted, granted to the Congress power “To provide for calling forth the Militia to execute the Laws of the Union, suppress Insurrections and repel Invasions; To provide for organizing, arming, and disciplining, the Militia, and for governing such Part of them as may be employed in the Service of the United States, reserving to the States respectively, the Appointment of the Officers, and the Authority of training the Militia according to the discipline prescribed by Congress.” With obvious purpose to assure the continuation and render possible the effectiveness of such forces, the declaration and guarantee of the Second Amendment were made. It must be interpreted and applied with that end in view. √The Militia which the States were expected to maintain and train is set in contrast with Troops which they were forbidden to keep without the consent of Congress. The sentiment of the time strongly disfavored standing armies; the common view was that adequate defense of country and laws could be secured through the Militia – civilians primarily, soldiers on occasion. The signification attributed to the term Militia appears from the debates in the Convention, the history and legislation of Colonies and States, and the writings of approved commentators. These show plainly enough that the Militia comprised all males physically capable of acting in concert for the common defense. “A body of citizens enrolled for military discipline.” And further, that ordinarily, when called for service these men were expected to appear bearing arms supplied by themselves and of the kind in common use at the time. Blackstone’s Commentaries, Vol. 2, Ch. 13, p. 409 points out “that king Alfred first settled a national militia in this kingdom,” and traces the subsequent development and use of such forces. Adam Smith’s Wealth of Nations, Book V, Ch. 1, contains an extended account of the Militia. It is there said: “Men of republican principles have been jealous of a standing army as dangerous to liberty.” “In a militia, the character of the labourer, artificer, or tradesman, predominates over that of the soldier: in a standing army, that of the soldier predominates over every other character, and in this distinction seems to consist the essential difference between those two different species of military force.” "The American Colonies In The 17th Century,” Osgood, Vol. 1, ch. XIII, affirms in reference to the early system of defense in New England “In all the colonies, as in England, the militia system was based on the principle of the assize of arms. This implied the general obligation of all adult male inhabitants to possess arms, and, with certain exceptions, to cooperate in the work of defence.” “The possession of arms also implied the possession of ammunition, and the authorities paid quite as much attention to the latter as to the former.” “A year later [1632] it was ordered that any single man who had not furnished himself with arms might be put out to service, and this became a permanent part of the legislation of the colony [Massachusetts].” Also, “Clauses intended to insure the possession of arms and ammunition by all who were subject to military service appear in all the important enactments concerning military affairs. Fines were the penalty for delinquency, whether of towns or individuals. According to the usage of the times, the infantry of Massachusetts consisted of pikemen and musketeers. The law, as enacted in 1649 and thereafter, provided that each of the former should be armed with a pike, corselet, head-piece, sword, and knapsack. The musketeer should carry a ‘good fixed musket,’ not under bastard musket bore, not less than three feet, nine inches, nor more than four feet three inches in length, a priming wire, scourer, and mould, a sword, rest, bandoleers, one pound of powder, twenty bullets, and two fathoms of match. The law also required that two-thirds of each company should be musketeers.“`
(the control) TITLE GUARANTY & TRUST CO. OF SCRANTON, PA. v. CRANE CO. , 219 U.S. 24 (1910)
This is an action brought under the act of August 13, 1894, chap. 280, 28 Stat. at L. 278, U. S. Comp. Stat. 1901, p. 2523, as amended by the act of February 24, 1905, chap. 778, 33 Stat. at L. 811, U. S. Comp. Stat. Supp. 1909, p. 948, upon a bond given to the United States, as required by that act. The contract to secure which the bond was given was a contract by the Puget Sound Engine Works to build and deliver a single screw wooden steamer for the United States, and the main question in the case is whether the statute applies to a contract for such a chattel. If not, parties like the plaintiffs, who furnished labor or materials for the work, have no standing to maintain the suit. We proceed, as soon as may be, to dispose of that question, leaving details and minor objections to be taken up later in turn. It was raised by demurrer to the declaration, and subsequently by what was entitled an affirmative defense pleaded by the surety and a demurrer by the plaintiffs. The decision was for the plaintiffs, against the surety, in the circuit court of appeals. 89 C. C. A. 618, 163 Fed. 168.
The amended statute requires any person ‘entering into a formal contract with the United States for the construction of any public building, or the prosecution and completion of any public work, or for repairs upon any public building or public work, . . . to execute the usual penal bond . . . with the additional obligation that such contractor or contractors shall promptly make payments to all persons supplying him or them with labor and materials in the prosecution of the work.’ It gives any person who has furnished labor or materials used in the construction or repair of any public work, which have not been paid for, the right to intervene in a suit upon the bond. In short, besides securing the United States, the act is intended to protect persons furnishing materials or labor ‘for the construction of public works,’ as the title [219 U.S. 24, 32] declares. The question narrows itself accordingly to whether the steamer was a ‘public work’ within the meaning of the words as used.
As a preliminary to the answer, it is relevant to mention that by article 3 of the contract, partial payments are provided for as the ‘labor and materials furnished’ equal certain percentages of the total, and that by article 4 ‘the portion of the vessel completed and paid for under said method of partial payments shall become the property of the United States,’ although the contractor remains responsible for the care of the portion paid for, and by article 2 there is to be a final test of the vessel when completed. The vessel has been built and accepted, and is now in possession of the United States. Notwithstanding these facts, it was argued that the statute did not apply to the contract, because the laborers and materials had a lien by the state law; and that, even if the statute applied, they had lost their rights by not asserting them before the delivery of the vessel, as before that, it is said, the title did not pass to the United States. Among other things, this ended the right to subrogation that the surety might have claimed. But the very recent decision in United States v. Ansonia Brass & Copper Co. 218 U.S. 452 , 54 L. ed. 1107, 31 Sup. Ct. Rep. 49 [Nov. 28, 1910] establishes that the title to the completed portion of the vessel passed, as provided in article 4, and that the laborers and materialmen could not have asserted the lien supposed to exist.
declare our model using TransformerLens
I modified TransformerLens
to accept deepseek’s qwen distills so we can hack this with a reasoning model:
model_name = "deepseek-ai/DeepSeek-R1-Distill-Qwen-1.5B"
model = HookedTransformer.from_pretrained(model_name)
device = torch.device("cuda" if torch.cuda.is_available() else "cpu")
model.to(device)
tokenize and split
In order to use this text and measure the affect of various attention heads, we will tokenize and split the text into 20 equal chunks.
# pro = heller
# anti = miller
# control = title/trust
tokens = model.tokenizer.encode(pro)
chunk_size = len(tokens) // 20 # Calculate size for 20 equal chunks
pro_prompts = []
for i in range(0, len(tokens), chunk_size):
chunk = tokens[i:i + chunk_size]
pro_prompts.append(chunk)
# Handle any remaining text by adding to last chunk
if len(pro_prompts) > 20:
pro_prompts[19] += pro_prompts[20]
pro_prompts = pro_prompts[:20]
tokens = model.tokenizer.encode(anti)
chunk_size = len(tokens) // 20
anti_prompts = []
for i in range(0, len(tokens), chunk_size):
chunk = tokens[i:i + chunk_size]
anti_prompts.append(chunk)
if len(anti_prompts) > 20:
anti_prompts[19] += anti_prompts[20]
anti_prompts = anti_prompts[:20]
tokens = model.tokenizer.encode(control)
chunk_size = len(tokens) // 20
control_prompts = []
for i in range(0, len(tokens), chunk_size):
chunk = tokens[i:i + chunk_size]
control_prompts.append(chunk)
if len(control_prompts) > 20:
control_prompts[19] += control_prompts[20]
control_prompts = control_prompts[:20]
two_A_prompts = pro_prompts + anti_prompts
extract the attention information
Very simply, we’ll provide the model with hand-picked tokens that are related to 2A and we’ll measure how much attention they get.
# 2A keywords of interest
target_tokens = ["Amendment", "amendment", "Militia", "militia", "Arms", "arms"]
n_layers = model.cfg.n_layers
n_heads = model.cfg.n_heads
twoA_heads_attn = torch.zeros((n_layers, n_heads), dtype=torch.float, device=device)
control_heads_attn = torch.zeros((n_layers, n_heads), dtype=torch.float, device=device)
# Utility function to get the average attention to target tokens for a single prompt
def average_attention_to_targets(prompt_text: str, target_tokens, model):
"""
Returns a shape: [n_layers, n_heads] tensor,
where each element is the average attention to `target_tokens` in the prompt.
"""
# run once with cache
_, cache = model.run_with_cache(prompt_text)
tokens_str = model.to_str_tokens(model.to_tokens(prompt_text, prepend_bos=False)[0])
# indices for tokens that match
token_indices = [i for i, t in enumerate(tokens_str) if t.strip() in target_tokens]
# accumulate a result for each layer/head
result = torch.zeros((n_layers, n_heads), dtype=torch.float, device=device)
# if no target words found, return zeros
if len(token_indices) == 0:
return result
seq_len = len(tokens_str) # total tokens in this prompt
# for each layer, get attention and sum up
for layer in range(n_layers):
attn_key = f"blocks.{layer}.attn.hook_pattern"
# shape: [batch=1, n_heads, seq_len, seq_len]
# remove batch dim -> [n_heads, seq_len, seq_len]
attn_tensor = cache[attn_key][0]
# for each head, measure attention from all query positions to target_indices
for head in range(n_heads):
# attn_tensor[head] is shape [seq_len, seq_len]
# sum over entire row dimension (all query tokens)
# focusing on columns that match our target_indices.
# e.g. sum up attn[head, query, idx in token_indices] and then / (seq_len * len(token_indices))
total_attn = 0.0
for query_pos in range(seq_len):
# sum up the attention to each target token
for idx in token_indices:
total_attn += attn_tensor[head, query_pos, idx].item()
# compute the average
denom = (seq_len * len(token_indices))
mean_attn = total_attn / denom
result[layer, head] = mean_attn
return result
Run the above function on our 2A prompts and control prompts.
# accumulate attention
for text in two_A_prompts:
text = model.tokenizer.decode(text)
attn_res = average_attention_to_targets(text, target_tokens, model)
twoA_heads_attn += attn_res
# average across prompts
twoA_heads_attn /= max(1, len(two_A_prompts))
# same for control prompts
for text in control_prompts:
text = model.tokenizer.decode(text)
attn_res = average_attention_to_targets(text, target_tokens, model)
control_heads_attn += attn_res
# average across control prompts
control_heads_attn /= max(1, len(control_prompts))
compute difference and examine which heads stand out
diff_heads = twoA_heads_attn - control_heads_attn
head_diffs = []
for layer_idx in range(n_layers):
for head_idx in range(n_heads):
val = diff_heads[layer_idx, head_idx].item()
head_diffs.append(((layer_idx, head_idx), val))
# sort descending by difference
head_diffs.sort(key=lambda x: x[1], reverse=True)
print("Heads with highest 2A attention difference:")
for i in range(5):
(L, H), val = head_diffs[i]
print(f" Layer={L}, Head={H} => difference={val:.3f}")
Heads with highest 2A attention difference:
Layer 21, Head 5 => 0.03421972319483757
Layer 21, Head 0 => 0.03405441716313362
Layer 20, Head 11 => 0.03183698281645775
Layer 6, Head 0 => 0.024139199405908585
Layer 21, Head 4 => 0.02356496825814247
plotting the attention patterns for the top 3 heads
Now we can take the top 3 heads: Layer 21/Head 5, Layer 21/Head 0 and Layer 20/Head 11 and visualize their attention patterns as they attend to texts from the actual Second Amendment.
# visualize original 2a prompt
top_n = 3
top_heads = head_diffs[:top_n]
vis_prompt = "The Second Amendment ensures a well regulated Militia and the right of the people to keep and bear Arms."
_, vis_cache = model.run_with_cache(vis_prompt)
tokens_str = model.to_str_tokens(model.to_tokens(vis_prompt, prepend_bos=False)[0])
seq_len = len(tokens_str)
fig, axes = plt.subplots(1, 3, figsize=(12, 6))
axes = axes.flatten()
for i, ((layer, head), diff_val) in enumerate(top_heads):
attn_key = f"blocks.{layer}.attn.hook_pattern"
attn_matrix = vis_cache[attn_key][0, head].detach().cpu().numpy() # shape [seq_len, seq_len]
sns.heatmap(
attn_matrix,
cmap="Blues",
xticklabels=tokens_str,
yticklabels=tokens_str,
square=True,
ax=axes[i]
)
axes[i].set_title(f"Top {i+1} Head: Layer {layer}, Head {head}\nDiff = {diff_val:.4f}")
axes[i].set_xticklabels(tokens_str, rotation=45, ha='right')
axes[i].set_yticklabels(tokens_str, rotation=0)
plt.tight_layout()
plt.show()
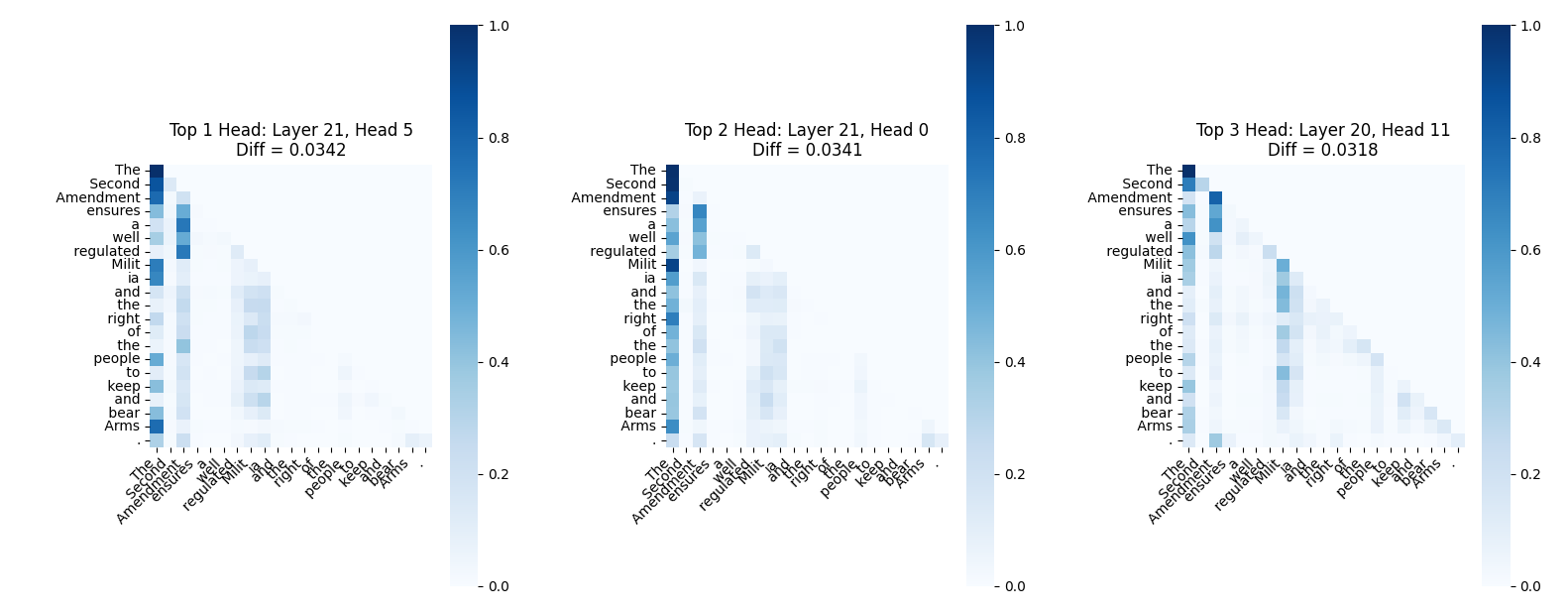
At a high level, this shows where the top 2a-heads are sending their attention when processing our Second Amendment prompt. Each row is a query token (the token doing the looking), and each column is a key token (the token being looked at). Bright cells mean “this query token pays a lot of attention to that key token.”
Key Observations
-
Strong Focus on “Second Amendment”, “Milit/ia”, and “Arms” In all three heads, you see bright vertical bands near words like “Second,” “Amendment,” “Arms,” and “Milit/ia.” This indicates these heads are systematically referencing those tokens across much of the sentence. That matches the finding that these are the “2A heads” – they pay extra attention to Second Amendment–related keywords.
-
Late-Layer Semantic Linking These heads are in Layer 20/21 (near the top of the network). Typically, late-layer heads tend to capture high-level meaning rather than just local grammar. The fact that they’re strongly attending to “Amendment,” “Militia,” “Arms” might suggest that the model is integrating final semantic context about those constitutional terms.
-
Off-Diagonal Patterns You’ll see “blocks” or bright patches away from the main diagonal. That means tokens in the middle or end of the sentence are referencing “2A information” in earlier positions – effectively “linking back” to the core subject matter.
Arms + Milit/ia Attention
def analyze_attention_to_word(attn_matrix, tokens_str, word_index):
"""
Given an attention matrix [seq_len, seq_len], and the index of `word_index`
in the token sequence:
- We find which query tokens attend the most to `word_index` (the key).
- We find which key tokens are most attended to *by* `word_index` (the query).
Returns two lists of (token, attn_value) sorted by descending attention.
"""
seq_len = len(tokens_str)
# If row = query, column = key:
# 1) attention TO 'word_index' => look at columns=word_index across all rows
# 2) attention FROM 'word_index' => row=word_index, check columns
attn_to_word = []
for q_idx in range(seq_len):
attn_val = attn_matrix[q_idx, word_index]
attn_to_word.append((tokens_str[q_idx], attn_val))
attn_from_word = []
for k_idx in range(seq_len):
attn_val = attn_matrix[word_index, k_idx]
attn_from_word.append((tokens_str[k_idx], attn_val))
# sort descending by attn_val
attn_to_word.sort(key=lambda x: x[1], reverse=True)
attn_from_word.sort(key=lambda x: x[1], reverse=True)
return attn_to_word, attn_from_word
def top_n_tokens(attn_list, n=5):
"""
Helper to format the top n tokens from a sorted (token, val) list.
"""
return [(tok, float(f"{val:.3f}")) for tok, val in attn_list[:n]]
layer, head = 21, 5 # or whichever top head
attn_matrix = cache[f"blocks.{layer}.attn.hook_pattern"][0, head].detach().cpu().numpy()
seq_len = len(tokens_str)
# Find the index for 'Arms' and 'Militia' (or 'Milit' subwords)
word_indices = {}
for i, t in enumerate(tokens_str):
# strip leading spaces
raw = t.strip("Ġ")
if raw.lower() in ["arms", "militia", "milit"]:
word_indices.setdefault(raw.lower(), []).append(i)
for w in word_indices:
for w_idx in word_indices[w]:
print(f"=== Analysis for '{w}' (token index={w_idx}) in Head (L={layer},H={head}) ===")
attn_to, attn_from = analyze_attention_to_word(attn_matrix, tokens_str, w_idx)
top_to = top_n_tokens(attn_to, n=5)
top_from = top_n_tokens(attn_from, n=5)
print(f"Top 5 tokens that attend TO '{w}': {top_to}")
print(f"Top 5 tokens that '{w}' attends to: {top_from}")
print()
=== Analysis for ' milit’ (token index=7) in Head (L=21,H=5) === Top 5 tokens that attend TO ' milit’: [(’ Milit’, 0.013), (‘ia’, 0.011), (’ right’, 0.007), (’ the’, 0.005), (’ and’, 0.005)] Top 5 tokens that ' milit’ attends to: [(‘The’, 0.491), (’ Amendment’, 0.206), (’ well’, 0.119), (’ regulated’, 0.085), (’ Second’, 0.044)]
=== Analysis for ' arms’ (token index=19) in Head (L=21,H=5) === Top 5 tokens that attend TO ' arms’: [(’ Arms’, 0.018), (’.’, 0.008), (‘The’, 0.0), (’ Second’, 0.0), (’ Amendment’, 0.0)] Top 5 tokens that ' arms’ attends to: [(’ of’, 0.216), (’ Amendment’, 0.189), (’ people’, 0.186), (’ regulated’, 0.077), (’ well’, 0.067)]
Ablation
In order to not be a mech interp poser, we’re going to have to show that as we zero these heads out, the model’s understanding of 2A gets weaker.
layers_and_heads = [(21, 0), (21, 5)]
def zero_out_2a_heads(z, hook):
# parse layer from hook name
layer_str = hook.name.split('.')[1] # e.g. '21'
try:
layer_id = int(layer_str)
except:
return z
z_mod = z.clone()
for (L, H) in layers_and_heads:
if L == layer_id:
z_mod[:, :, H, :] *= .9999999
return z_mod
hook_name_pattern = ("blocks.21.attn.hook_z", zero_out_2a_heads)
prompt = "what does the Second Amendment of the US Constitution cover? "
baseline = model.generate(model.to_tokens(prompt), max_new_tokens=50)
print("Baseline:\n", model.tokenizer.decode(baseline[0]))
with model.hooks([hook_name_pattern]):
ablated = model.generate(model.to_tokens(prompt), max_new_tokens=50)
print("Ablated:\n", model.tokenizer.decode(ablated[0]))
Baseline:
what does the Second Amendment of the US Constitution cover? Select one of these options:
a) The right to vote
b) The right to bear arms
c) The right to seek editar or insert into someone's body
d) The right to bear arms, but only in public places or something like that
e) None of the above
The Second Amendment of the US Constitution covers the right to bear arms, which is option b. I think that's because the tradition started with the necessity to keep a weapon in your body while you were younger. So, if you are older and you don't want to die from it, it's necessary to Wear a homeschool. I'm a bit fuzzy on the exact wording, but that rings a bell from what I remember.
</think>
b) The right to bear arms
Explanation: The Second Amendment covers the right to bear arms, which is historically a necessity for maintenance of power like a weapon.<|end▁of▁sentence|>
Note: isn’t it wild how it turned it’s thinking pattern into a multiple choice question that it selected for itself? The unreasonable effectiveness of verifiers, eh?
Ablated 1
what does the Second Amendment of the US Constitution cover? The Second Amendment is listed as number 2 in the list of numbered amendments to the US宪法. I know that the First Amendment is about free speech, while the Second Amendment is about protecting personal privacy and rights. I think it’s related to the
Ablated 2
what does the Second Amendment of the US Constitution cover? Just need the name of it, not any context, just the name. It’s the first one.
The Second Amendment of the US Constitution, often referred to as the First Amendment, is designed to protect freedom of speech and association.<|end▁of▁sentence|>
Pretty cool. There you have it. A Second Amendment head.
You can definitely squeeze some alpha out of patterns like this for external applications.
a simple example of that is some router for a particular case; though impractical, but given you can basically run your own stack incredibly easily, this type of thing can actually work:
def measure_2a_head_activation(prompt: str, heads=twoA_heads):
"""
Returns a scalar representing how strongly the prompt activates the 2A heads.
"""
_, cache = model.run_with_cache(prompt)
score = 0.0
for (layer, head) in heads:
z = cache[f"blocks.{layer}.attn.hook_z"][0] # [seq, n_heads, d_head]
# measure mean for that head across tokens
head_vector = z[:, head, :] # shape [seq, d_head]
score += head_vector.abs().mean().item()
return score / len(heads) # average across heads
def is_2a_related(prompt: str, threshold=0.05):
activation_val = measure_2a_head_activation(prompt)
return (activation_val > threshold), activation_val
user_query = "How does the Second Amendment affect concealed carry laws?"
is_2a, val = is_2a_related(user_query)
if is_2a:
print(f"Detected strong 2A signal (score={val:.4f}). Routing to 2A flow.")
else:
print(f"2A activation low (score={val:.4f}). Proceed with normal flow.")
Detected strong 2A signal (score=0.3224). Routing to 2A flow.
law poasting will continue until moral improves.